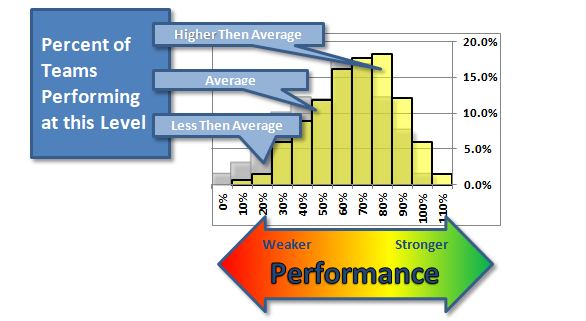
Question: “What’s the difference between a scientist and a talk show host?”
Answer: “A scientist keeps looking, even after they find an answer they like.”
Ultimate has way too many talk show hosts and not nearly enough scientists. Loud and uninformed opinions abound. There seem to be claims of unfairness sprinkled throughout the sport. Some groups feel they are being treated differently without providing supporting cause or reason. It turns out that topic is well beyond the scope of this paper. This paper will attempt to do something related but at a more fundamental level.
This paper is an attempt to make the underlying facts visible. Only after the underlying causal relationships are recognized, acknowledged & accepted by all parties involved, can effective solutions be implemented.
Let’s get going.
Team Division Differences in Ultimate
As I said above, this paper is somewhat limited in its scope. It will look into a small piece of the team performance puzzle. This paper will address whether there are performance differences in teams by their division. It will do this by applying some respected scientific methodologies to readily available data. Everything here is re-creatable by the reader.
Testing Methodology
The best testing methodology is to apply some proven statistical analysis tools to the recorded game scores. In general, statistical analysis takes advantage of some astute observations about the natural world that have been made down through the years. Early uses of statistical analysis techniques were by gamblers looking for an edge and governments deciding on tax rates. Out of this murky past a science evolved. In the ensuing years, more sophisticated tools and processes have developed to answer all manner of statistical questions.
Science has learned that the best way to prove something is by proposing a Hypothesis and then testing its validity against something called the Null Hypothesis.
Hypothesis & Null Hypothesis
Hypothesis
An idea proposed for the sake of argument so that it can be tested against populations (data) to see if it might be true.
Null Hypotheses
There is no statistical difference between the two populations being studied.
It turns out in real science; you can’t prove something right unless it is also possible to prove it wrong. Hypotheses need to stated in such a way that they can be proved wrong. So, what we are going to do is to create a Null Hypothesis and prove that wrong. Crazy as it sounds, we’re going to prove something right by proving the opposite is wrong.
The hypothesis being offered in this article is “There is a Difference in the Performance of Elite Teams when Evaluated by Division.” In our case, this would make our Null Hypothesis “There is No Difference in the Performance of Elite Teams when Evaluated by Division.”
Hypothesis Testing
We test the hypothesis by comparing a test population (Open teams, Mixed teams & Women’s teams) against a reference population (everyone) and determine the likelihood of the differences being due to random variations. There are a number of tests available to determine the likelihood the difference in measured values is random.
Deciding on the analysis tool requires understanding the form of the data. A quick look at the raw data here indicates the data is distributed but isn’t strictly Normal and we will end up using a more general test for data validation. In this case, the test applied is the “Two sample, unpaired, two tail Student’s t-test”.
Two sample, unpaired, two tail Student’s t-test
“Student” was the pen name of one William Sealy Gosset (1876-1937). Gosset, employed by the Guinness Brewery in Dublin, was tasked with coming up with a statistical way to monitor the quality of the Guinness stout. Since the Guinness Brewery forbids any of its chemists from publishing, Gosset decided to publish anyway; under the pseudonym Student. The test he created was quickly embraced by other researchers around the world. The Student’s t-test became an important part of every researcher’s tool kit.
t-test details:
Two sample Comparing two groups of data
Unpaired The values are not related to one another
(i.e. – no game score affects another game score)
Two tailed The data values are clustered in the middle and tail off towards the ends
The t-test produces a single number describing the likelihood that the difference between the two samples is due to random variations. The number we’re looking for is 0.05 (or 5%). That is, if the t-test score is less than 0.05, the samples are considered different.
Another Statistical Tool
If the data proves to be believable, other tools can then be applied to better understand the characteristic differences and what they mean. The most common follow up analysis tool would be the Mean & Standard Deviation calculations.
Mean & Standard Deviation
For those of you who don’t live in a statistical world, here’s a quick explanation of the terms Mean & Standard Deviation; as well as when they can be used.
Mean
Mean (Average) is just all the values added together and then divided by the number of values. I.e.; if a team plays 10 games and scores a total of 131 goals; their mean (average) score is (131 / 10) or 13.1 goals per game.
Standard deviation (Std Dev)
Standard Deviation is a measure of how consistent the team was in achieving the 131 goals. Teams can reach a total number of points in many different ways. In the case of 131 points scored, below are 3 teams (A, B & C). Each one of those teams scored 131 goals in 10 games in a different way
Team | 1 | 2 | 3 | 4 | 5 | 6 | 7 | 8 | 9 | 10 | Total | Mean | Std Dev |
A | 15 | 15 | 15 | 15 | 15 | 15 | 15 | 15 | 11 | 0 | 131 | 13.1 | 4.77 |
B | 14 | 13 | 13 | 13 | 13 | 13 | 13 | 13 | 13 | 14 | 131 | 13.1 | 0.32 |
C | 14 | 14 | 14 | 14 | 14 | 13 | 12 | 12 | 12 | 12 | 131 | 13.1 | 0.99 |
Note:
If this was a tournament, team B probably finished higher than team A or team C because of their more consistent play. So….a high Mean and a low Standard Deviation are desirable. Just who cares about high Means and low Standard Deviations? Coaches, Tournament Selection Committees and Media Marketing Directors care.
Alas, the Standard Deviation can only be strictly applied to a Standard Normal Distribution. If the Mean is too far from the physical middle of the data, the Standard Deviations can extend off the end of the data. In the above example, Team A has a mean score of 13.1 and a Standard Deviation of 4.77. This means; Teams A has a typical game score somewhere between 8.33 and 17.87 points. 17.87 points, not bad for a game to 13. Still, the Standard Deviation number is a very good indication of team consistency. In fact, we can look at the Standard Deviation in a slightly different way and use something called the “Three Sigma Rule of Thumb”.
Three Sigma Rule of Thumb.
The “Three Sigma Rule of Thumb” is a well-used heuristic approach to looking at distributed data. The word Sigma is shorthand for 1 Standard Deviation. The test is more formally referred to as the “68-95-99.7 Rule.” In this approach, the data in question is grouped by the by the number of teams expected to be in each Standard Deviation level. This rule says any team value less than 1 Sigma from the Mean is considered average. Any team value greater than 1 Sigma but less than 2 Sigma is considered above or below average depending on the direction. Anything outside of a 2 Sigma distance is considered as under or over performing. Here, the Three Sigma Rule will be used to compare a specific Team Division population against the complete population.
Histograms
The Three Sigma Rule lists how many teams fit into each Sigma level. Histograms provide a visual presentation of Team Division performance against the total population in greater detail.
Statistical Analysis Tools Summary
To recap, the analysis will yield 4 things
- Histograms of Team Division against the composite
- t-test for significance against the composite
- Mean & Standard Deviation within the test population
- 3 Sigma comparison to the total population
Team division considered
Three team divisions were studied; Open, Mixed & Women’s.
Open – No gender restrictions are in place
Mixed – A set number of each gender as per tournament rules.
Women – Players who self-identify as women.
Assumptions
One assumption being made in this paper is that elite level ultimate is the role model for the sport. Another assumption being made is that the upper tier USAU Triple Crown Tour represented by a defined set of tournaments is a fair representation of elite level ultimate. USAU focuses on collecting scores and individual fantasy stats and not on measurements of any useful team performance parameters so data from USAU is somewhat limited. Several performance parameters will need to be inferred from the available data.
Triple Crown Tour (TCT)
Moving forward with the Elite Ultimate data, I selected game scores from the following tour events:
- Pro-Elite Challenge
- Select Flight Invite
- US Open Club Championships
- Elite-Select Challenge
- Nationals
I was able to access TCT data for 2014, 2015, 2016, 2017 & 2018 on the USAU website. 2013 data was found on UltiArchieve.com.
I did not include Sectionals or Regionals data. The data collected included:
Year | Mixed | Open | Women | Yearly Total |
2013 | 201 | 194 | 201 | 596 |
2014 | 184 | 194 | 197 | 575 |
2015 | 246 | 253 | 234 | 733 |
2016 | 255 | 260 | 249 | 764 |
2017 | 271 | 270 | 271 | 812 |
2018 | 261 | 262 | 261 | 784 |
All Years | 1,418 | 1,433 | 1,413 | 4,264 |
Data Used
When assessing performance, a single overarching parameter is needed. This parameter must be calculated from objectively measured data.
Data Used:
- Team Division
- Year
- Winning Score
- Losing Score
- Data Inferred
- Game to Score
- Data Discarded
- Team Name
- Tournament Name
- Brackets
- Placements
Data Desired but not Available:
- Actual Game Time Duration
- Time to Soft Cap
- Time to Hard Cap
Analysis Parameters
Since there were only 3 measured items and one inferred item, those items needed to be combined to create meaningful analysis parameters. The following 5 parameters were created.
Winning Performance Index
WPI=(“Winning Score”)/(“Game to”)
Losing Performance Index
LPI=(“Losing Score”)/(“Game to”)
Aggregate Performance Index (API)
API=(“WPI”+”LPI”)/2
Score Differential Index (SDI)
The SDI is a measurement of game closeness.
SDI=1-“Game Diff”-1/(“Winning Score”)
Spectator Viewing Index (SVI)
An exciting game is a high scoring, close game.
SVI=(API *”SDI”)
An explanation of the SVI:
I’ve included the SVI parameter & chart because of the increasing media exposure the game is seeking/achieving. When games & tournaments were played for the benefit of the players, family & friends and were funded strictly by team fees, this was unimportant. Now, with the addition of media exposure, advertising and with sponsor prize money being awarded, the entertainment & marketing value of a game becomes increasingly important.
Processing the data
Processing the data for presentation was somewhat complicated because some TCT tournament games were to 13 and some were to 15 points. Direct visual comparison wasn’t possible. To compensate for this, I normalized the data and resampled.
The Charts
The data on each chart set depicts several interpretations of a single parameter with respect to team division.
Histograms
The first row displays histograms of each team division’s performance overlaid on the composite of the three team divisions. Bright colors indicate exceeding the average number of teams and dark colors indicate a below average number of teams. In general, exceeding the composite high and to the right is better than exceeding low and to the left.
Charts will always be presented highest average to the left and lowest average to the right for the displayed parameter regardless of team division.
Histogram Legend
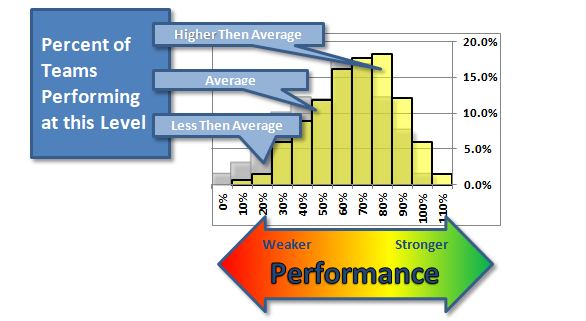
Over Performing the Composite
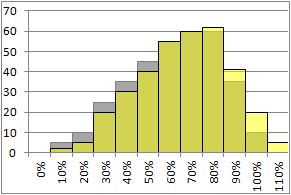
The above chart is indicative of a team overperforming against the Composite.
Under Performing the Composite
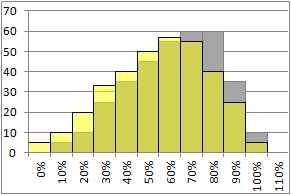
This chart illustrates a team underperforming when compared to the composite.
Average Rankings
The team divisions are ranked highest to lowest (including the composite of all teams) by Mean (average) value. Also included are the Standard Deviation and number of games included.
t-test for Significance
All combinations of t-test populations were evaluated and ranked
3 Sigma Ranking
Each team division is shown as it fits in with the 3 Sigma parameter thresholds.
Aggregate Performance Index (API)
API = ( WPI + LPI ) / 2
The API represents the overall quality of the game. The higher the API; the more total points scored in the game.
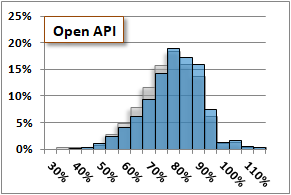
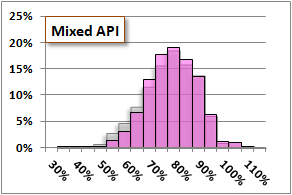
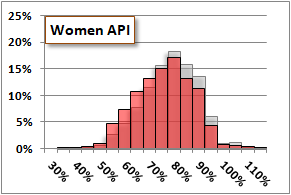
Average Rankings
Rank | Team Division | Average | Std Dev | # Games |
1 | Open | 82.64% | 9.7% | 1,432 |
2 | Mixed | 79.92% | 9.8% | 1,418 |
3 | Composite | 79.75% | 10.6% | 4,263 |
4 | Women | 76.67% | 11.3% | 1,413 |
t-test for Significance (API)
Rank | Combination | t Score | Statistically Significant |
1 | Open – Women | 1.25E-49 | Yes |
2 | Open – Composite | 8.91E-21 | Yes |
3 | Composite – Women | 4.28E-19 | Yes |
4 | Mixed – Women | 5.06E-16 | Yes |
5 | Open – Mixed | 3.31E-13 | Yes |
6 | Mixed – Composite | 5.31E-01 | No |
3 Sigma Rule of Thumb When Compared to the Composite Average
Team Division | % Over Performing | % Above Average | % Average | % Below Average | % Under Performing |
Open | 4.19% | 19.34% | 70.53% | 4.96% | 0.98% |
Mixed | 2.75% | 14.25% | 73.27% | 8.67% | 1.06% |
Women | 2.19% | 11.11% | 65.04% | 17.48% | 4.18% |
Winning Performance Index (WPI)
WPI = “Winning Team Score” / “Game to”
The WPI represents the performance of the winning team. The higher the WPI, the more points scored by the winning team.
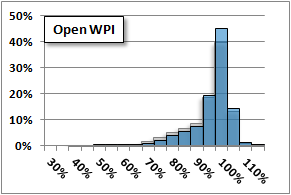
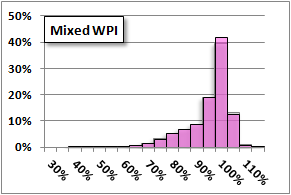
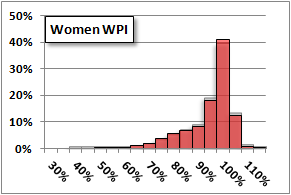
Average Rankings
Rank | Team Division | Average | Std Dev | # Games |
1 | Open | 97.09% | 7.07% | 1,432 |
2 | Composite | 95.14% | 8.83% | 4,263 |
3 | Mixed | 94.32% | 8.97% | 1,418 |
`4 | Women | 93.98% | 9.89% | 1,413 |
t-test for Significance
Rank | Combination | Score | Statistically Significant |
1 | Open – Women | 1.40E-21 | Yes |
2 | Open – Mixed | 1.06E-18 | Yes |
3 | Open – Composite | 3.25E-16 | Yes |
4 | Composite – Women | 9.34E-05 | Yes |
5 | Composite – Mixed | 4.19E-03 | Yes |
6 | Mixed – Women | 3.31E-01 | No |
3 Sigma Rule of Thumb When Compared to the Composite Average
Team Division | % Over Performing | % Above Average | % Average | % Below Average | % Under Performing |
Open | 4.61% | 67.67% | 23.39% | 3.84% | 0.49% |
Mixed | 2.89% | 55.85% | 29.69% | 10.72% | 0.85% |
Women | 2.41% | 58.95% | 24.70% | 12.24% | 1.70% |
Losing Performance Index (LPI)
LPI = “Losing Points Scored” / “Game to”
The LPI represents the performance of the losing team. The higher the LPI, the more points scored while still losing.
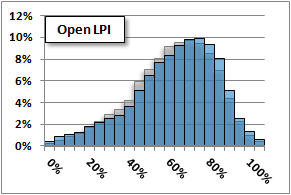
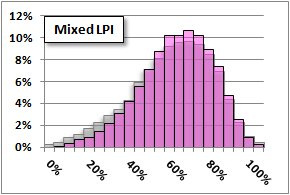
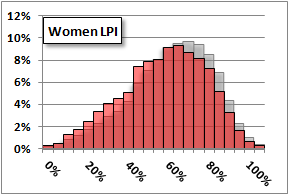
Average Rankings
Rank | Team Division | Average | Std Dev | # Games |
1 | Open | 68.19% | 17.02% | 1,432 |
2 | Mixed | 65.52% | 16.63% | 1,418 |
3 | Composite | 64.37% | 18.21% | 4,263 |
4 | Women | 59.36% | 19.70% | 1,413 |
t-test for Significance
Rank | Combination | Score | Statistically Significant |
1 | Open – Women | 2.26E-36 | Yes |
2 | Mixed – Women | 4.84E-19 | Yes |
3 | Composite – Women | 5.52E-17 | Yes |
4 | Open – Composite | 9.81E-13 | Yes |
5 | Open – Mixed | 3.03E-05 | Yes |
6 | Mixed – Composite | 2.62E-02 | Yes |
3 Sigma Rule of Thumb When Compared to the Composite Average
Team Division | % Over Performing | % Above Average | % Average | % Below Average | % Under Performing |
Open | 5.38% | 16.41% | 66.20% | 11.31% | 0.70% |
Mixed | 4.09% | 12.48% | 69.04% | 13.61% | 0.78% |
Women | 3.04% | 8.85% | 61.15% | 23.28% | 3.68% |
Score Differential Index (SDI)
SDI = 1 – (“Game Diff” – 1) / “Winning Score”
The SDI represents the closeness of the games. The higher the SDI, the closer the game.
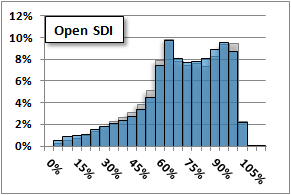
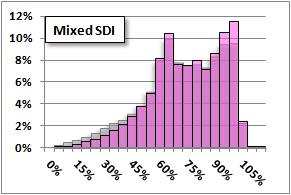
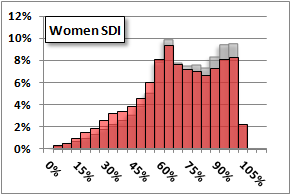
Average Rankings
Rank | Team Division | Average | Std Dev | # Games |
1 | Open | 78.00% | 17.53% | 1,432 |
2 | Mixed | 77.68% | 18.29% | 1,418 |
3 | Composite | 75.77% | 19.39% | 4,263 |
4 | Women | 71.61% | 21.49% | 1,413 |
t-test for Significance
Rank | Combination | Score | Statistically Significant |
1 | Open – Women | 6.54E-18 | Yes |
2 | Mixed – Women | 8.88E-16 | Yes |
3 | Composite – Women | 1.25E-10 | Yes |
4 | Open – Composite | 4.81E-05 | Yes |
5 | Mixed – Composite | 9.28E-04 | Yes |
6 | Open – Mixed | 5.99E-01 | No |
3 Sigma Rule of Thumb When Compared to the Composite Average
Team Division | % Over Performing | % Above Average | % Average | % Below Average | % Under Performing |
Open | 46.09% | 1.96% | 43.30% | 7.96% | 0.70% |
Mixed | 44.36% | 1.83% | 42.17% | 10.93% | 0.71% |
Women | 35.53% | 1.84% | 41.68% | 17.41% | 3.54% |
Spectator Viewing Index (SVI)
SVI = SDI * API
The SVI represents the whether the game was close with lots of points scored. The higher the SVI, the more exciting the game is to watch.
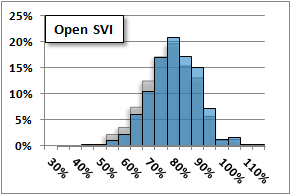
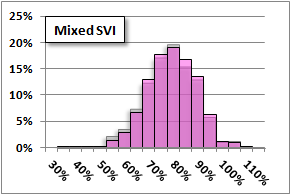
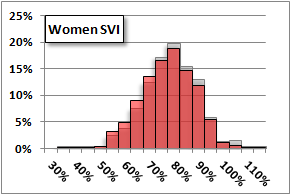
Average Rankings
Rank | Team Division | Average | Std Dev | # Games |
1 | Open | 65.70% | 19.98% | 1,432 |
2 | Mixed | 63.13% | 19.58% | 1,418 |
3 | Composite | 61.79% | 20.91% | 4,263 |
4 | Women | 56.49% | 22.02% | 1,413 |
t-test for Significance
Rank | Combination | Score | Statistically Significant |
1 | Open – Women | 8.63E-31 | Yes |
2 | Mixed – Women | 3.57E-17 | Yes |
3 | Composite – Women | 3.07E-15 | Yes |
4 | Open – Composite | 3.46E-10 | Yes |
5 | Open – Mixed | 6.23E-04 | Yes |
6 | Mixed – Composite | 2.68E-02 | Yes |
3 Sigma Rule of Thumb When Compared to the Composite Average
Team Division | % Over Performing | % Above Average | % Average | % Below Average | % Under Performing |
Open | 3.42% | 18.30% | 69.34% | 8.24% | 0.70% |
Mixed | 1.76% | 14.88% | 70.73% | 11.85% | 0.78% |
Women | 1.56% | 10.26% | 65.32% | 19.18% | 3.68% |
More Summary Information
Looking at the data a bit more produces the following summaries:
Measurement | Open | % | Mixed | % | Women | % | |
*Winning Score (avg) | 12.6 | 12.2 | 11.95 | ||||
*Losing Score (avg) | 8.8 | 8.5 | 7.48 | ||||
*Score Margin (avg) | 3.7 | 3.7 | 4.5 | ||||
*Total Points (avg) | 21.4 | 20.7 | 19.43 | ||||
Games Capped | 36.5 | 39% | 46.7 | 48% | 43.8 | 47% |
*Based on a game to 13.
Conclusion
The null hypothesis “There is no Difference in the Performance of Elite Teams when Evaluated by Division” is rejected. There is a statistically significant difference in performance when assessing Elite Ultimate teams by their division.
For some of the secondary comparisons, the analysis did not yield a statistically significant difference.
- For Mixed vs. The Composite, Aggregate Performance Index (API) could not be distinguished with sufficient confidence.
- For Mixed vs. Open teams, Relative point differentials (SDI) could not be distinguished with sufficient confidence.
- For Women vs. Mixed teams, the Winning Performance Index (WPI) could not be distinguished with sufficient confidence.
Bonus Information
TCT Parameters by Year
The above study concluded that there is a statistically significant difference in elite team performance when differentiated by division. The question next becomes how long has this been going on? The two following charts breakdown those differences by year.
API, WPI & LPI by Year
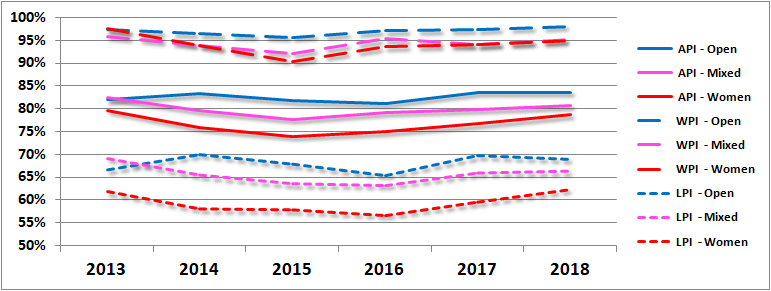
SDI & SVI by Year
USAU 2018 Nationals Comparisons
I was pursuing some research on a slightly different topic. This other topic, for a different article, was “How Do Team Skills Change at Each Age Level?” To explore that question, I transcribed all 2018 USAU game data for all National Championships at every level of play and produced this chart.
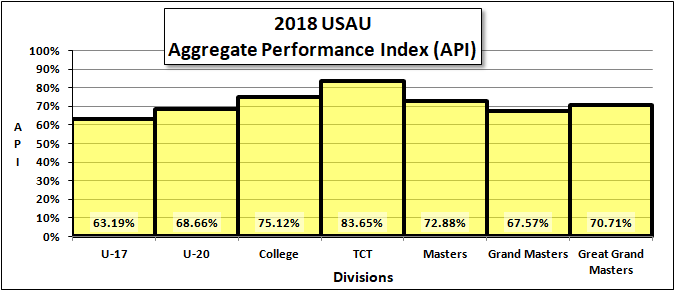
This chart was pretty much as expected; except for that “Grand Masters” dip. This dip caught my attention and aroused my curiosity. I wondered if there was a team division component to the data. So I took that data and further subdivided it by team division and got this.
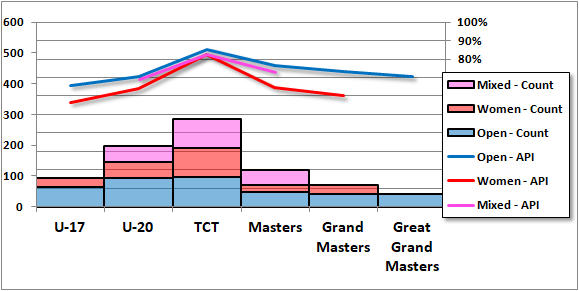
This chart shows team division performance differences across all USAU age divisions. The data does show the reason for the “Grand Masters” dip in API values. More importantly, in my opinion the division difference trends were unexpected considering the initiatives put into place recently by USAU. The unexpected starting point differences (U-17) bring into question the validity of the U-17 data. The data may just be a statistical anomaly stemming from an off year in the youth game or even poor tournament conditions. To answer that question, I went back and transcribed every national level youth game I could find. USAU was helpful; even to the point of sending me photocopied tournament results for data not available online. This is what I was able to find.
Youth Ultimate at the National Level
Youth Ultimate has evolved over the years, starting with some high school teams getting together in 1988 and ending up with the YCC extravaganza we see today. Here’s a quick recap of events:
Year | Level | Open
Games |
Mixed
Games |
Women
Games |
Total
Games |
||
1988 | UPA | HS | High School | Unknown | Unknown | ||
2004 | UPA | HS | High School | 43 | 40 | 83 | |
2005 | UPA | YCC | U-19 | 24 | 20 | 9 | 53 |
2006 | UPA | YCC | U-19 | 23 | 20 | 14 | 57 |
2007 | UPA | YCC | U-19 | 24 | 22 | 21 | 67 |
2008 | UPA | YCC | U-19 | 24 | 21 | 13 | 58 |
2009 | UPA | YCC | U-19 | 24 | 10 | 23 | 57 |
2010 | USAU | YCC | U-19 | 31 | 24 | 15 | 70 |
2011 | USAU | YCC | U-19 | 61 | 24 | 22 | 107 |
2012 | USAU | YCC | U-16 | 23 | 23 | ||
2012 | USAU | YCC | U-19 | 38 | 24 | 15 | 77 |
2013 | USAU | YCC | U-16 | 26 | 26 | ||
2013 | USAU | YCC | U-19 | 56 | 22 | 24 | 102 |
2014 | USAU | YCC | U-16 | 39 | 6 | 45 | |
2014 | USAU | YCC | U-19 | 70 | 31 | 32 | 133 |
2015 | USAU | YCC | U-16 | 47 | 24 | 71 | |
2015 | USAU | YCC | U-19 | 91 | 47 | 47 | 185 |
2016 | USAU | YCC | U-16 | 54 | 28 | 82 | |
2016 | USAU | YCC | U-19 | 106 | 52 | 49 | 207 |
2017 | USAU | YCC | U-17 | 66 | 33 | 99 | |
2017 | USAU | YCC | U-20 | 100 | 44 | 52 | 196 |
2018 | USAU | YCC | U-17 | 63 | 29 | 92 | |
2018 | USAU | YCC | U-20 | 93 | 52 | 51 | 196 |
TOTALS | 1126 | 413 | 547 | 2086 |
There were some Juniors level tournaments prior to 2004, but no information was available. Here’s the summary chart of available Youth Performance data through the years.
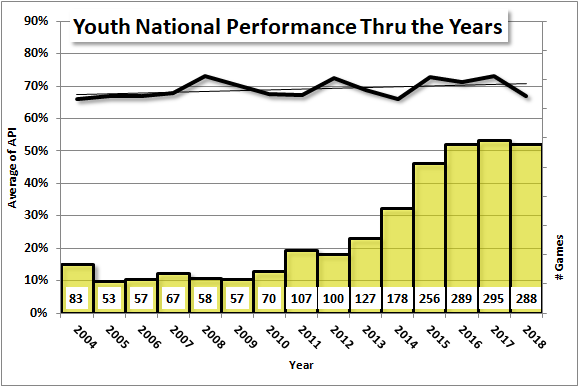
The histogram at the bottom of the chart illustrates the increase in the number of teams & games played at the youth national tournaments; from 2005’s low of 28 teams & 53 games to 2017’s 70 teams & 295 games. This chart held another surprise as well. I was not expecting to see the low advances in the quality of play in youth Ultimate over the years; a 0.24% growth rate over 14 years, but that’s for another article. But I digress, here’s the team division breakdown for the youth data.
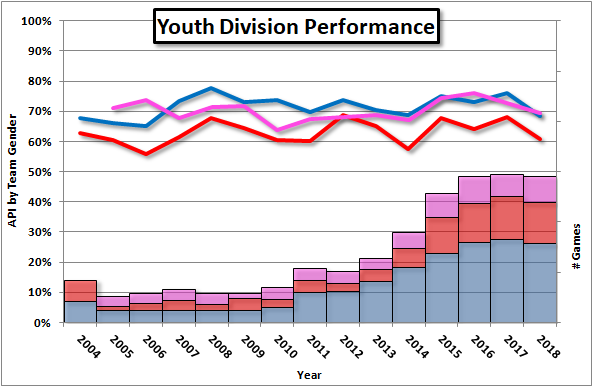
Average Rankings
Rank | Team Division | Average | Std Dev | # Games |
1 | Open | 72.41% | 11.55% | 1083 |
2 | Mixed | 70.72% | 11.38% | 413 |
3 | Composite | 69.99% | 11.99% | 2003 |
4 | Women | 64.22% | 11.47% | 507 |
t-test for Significance
Rank | Combination | Score | Statistically Significant |
1 | Open – Women | 5.86E-37 | Yes |
2 | Composite – Women | 2.33E-22 | Yes |
3 | Mixed – Women | 3.98E-17 | Yes |
4 | Open – Composite | 4.62E-08 | Yes |
5 | Open – Mixed | 1.07E-02 | Yes |
6 | Mixed – Composite | 2.39E-01 | Yes |
The data passes the t-test for significance. The youth team division differences are real and not a statistical aberration due to random variations.
2nd Conclusion
Statistically significant performance differences exist in youth Ultimate at the national level when evaluated by team division.
Comments Policy: At Skyd, we value all legitimate contributions to the discussion of ultimate. However, please ensure your input is respectful. Hateful, slanderous, or disrespectful comments will be deleted. For grammatical, factual, and typographic errors, instead of leaving a comment, please e-mail our editors directly at editors [at] skydmagazine.com.